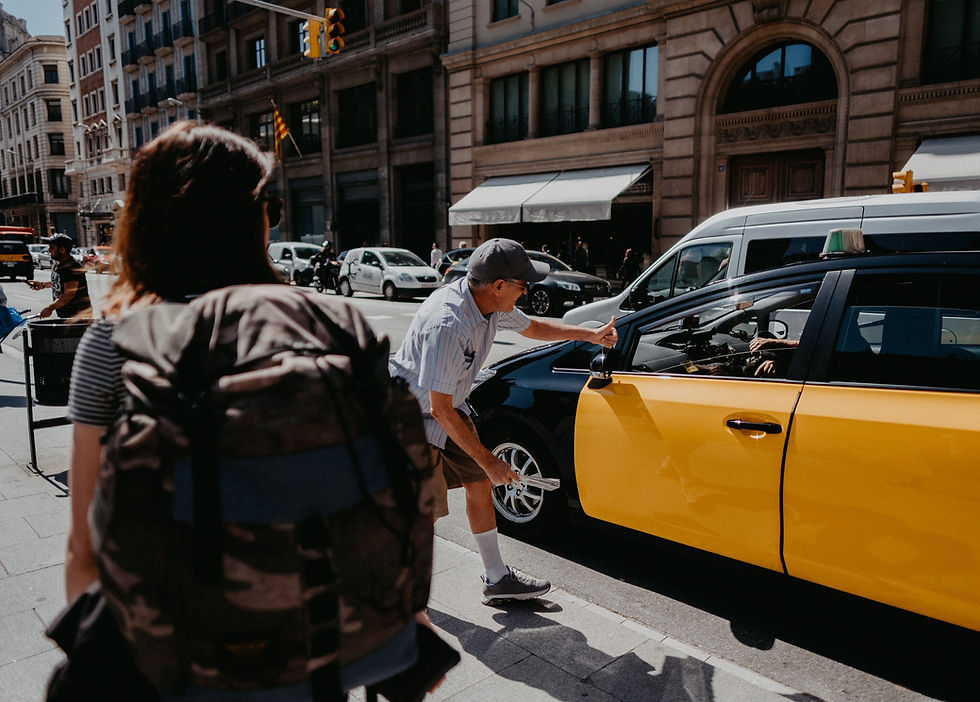
Ride-Hailing App
AI Integration Project
An AI integration project for a leading ride-hailing platform that enhanced user-driver matching, personalized in-app experiences, and boosted customer retention through intelligent promotions and behavioral insights.
client profile:
a leading Southeast Asian ride-hailing company with millions of users and thousands of active drivers across multiple metro cities. While the app had strong user traffic, it lacked advanced AI-driven personalization to improve user-driver matching, user engagement, and retention.
this project showcases how Crefel Technology Solutions successfully integrated artificial intelligence (AI) across the platform to analyze behavioral patterns, deliver personalized content, and drive long-term loyalty — resulting in measurable improvements in conversion rates, driver utilization, and customer retention.
PROJECT BRIEF
This complex AI integration project required end-to-end strategic consulting, agile model development, and robust testing. Crefel’s multidisciplinary approach—blending AI expertise, behavioral science, and full-stack engineering—enabled the ride-hailing app to transform user experience and ROI.
The project stands as a benchmark for what’s possible when technology meets customer intelligence at scale.


Phase 1: Discovery and Data Strategy (2 months)
• Crefel conducted an extensive audit of the platform’s data structure, ride history, app behavior logs, and ad placement metrics.
• Key gaps were identified: fragmented user behavior data, generic ad serving, and low repeat usage among new users.
• Designed a centralized behavioral analytics pipeline to fuel real-time AI models.
Phase 2: AI Model Development & System Integration (4 months)
Developed and deployed:
• A real-time matching algorithm that considers time of day, location, historical behavior, and user urgency to pair riders with the most relevant drivers.
• An ad-serving engine using contextual and behavioral tagging to increase ad click-through rates (CTR).
• A retention and loyalty engine that segments users and deploys targeted offers, cashback, and loyalty perks.
Phase 3: Testing, Optimization & Launch (3 months)
• A/B tested different model variations on select user groups.
• Measured lift in KPIs across conversion, app usage frequency, and trip booking rate.
• Soft launch followed by full roll-out across all cities.
solution:
To address the client’s need for smarter engagement and operational efficiency, Crefel implemented an end-to-end AI solution that transformed how users were matched, engaged, and retained within the app.
AI-Driven Matching Algorithm
Used deep learning to create predictive user profiles. Factored in rider preferences, cancellation behavior, and urgency sensitivity to match with the most appropriate driver based on driver availability and rating.
Predictive Ad Engine
Shifted from demographic-based ads to interest-based ads.
AI selected ad placements within the app based on real-time behavioral cues (e.g. browsing promos, time spent on app screens).
Loyalty & Offers Personalization Engine
Created smart retention segments: “frequent riders,” “churn risks,” “promo-driven,” and “new users.”
Delivered adaptive discounts, badges, and ride milestones to maximize repeat bookings and long-term engagement.
solutions:
Key features and results

Behavioral Mapping
Captured
behavioral
signals from
17M
app sessions
per month
AI-powered matching
improved ETA accuracy by
12%
and reduced ride
cancellations by 18%.
Personalized Promotions: Email/SMS/in-app offers personalized to individual ride history and usage frequency.
Ad Engagement AI: Contextual ad targeting increased CTR by 27% over generic ads.
Outcomes
AI-driven ride
suggestions
boosted ride
bookings by
21%
in targeted city
clusters during
peak hours.
Retention among
first-time users
increased by
38%
over a 90-day period,
driven by personalized
loyalty offers and
milestone-based
gamification.
Operational Efficiency & Driver Utilization
-
Driver idle time dropped by 15%, and average match time per ride decreased from 42 seconds to 28 seconds.
-
More relevant matching reduced driver complaints and improved ratings by 0.3 stars on average.
Revenue Uplift
-
Targeted in-app promotions and cross-sell ad inventory led to 9% increase in revenue per user (ARPU).